About Machine Learning: Unlocking the Future of Business
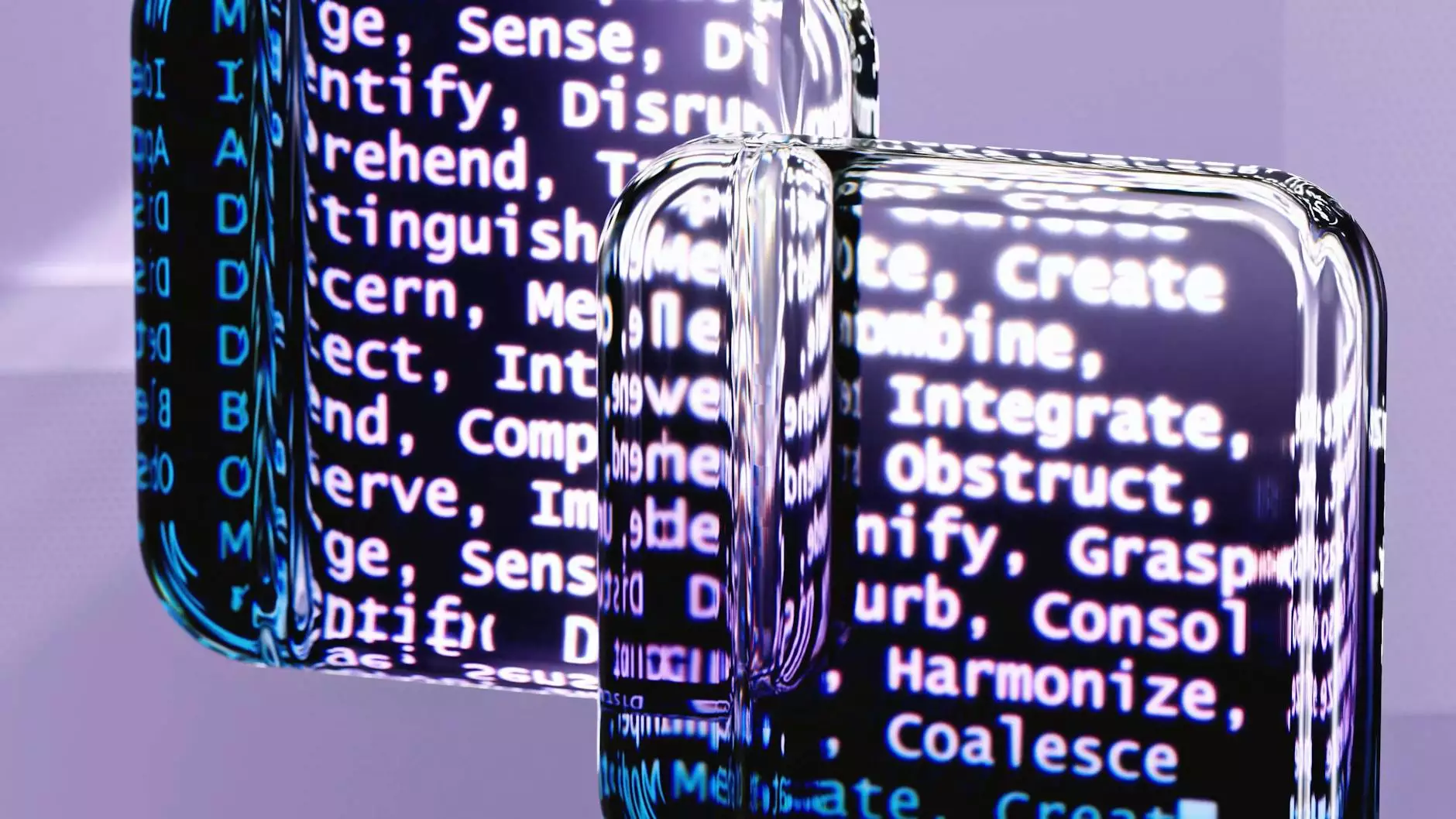
Machine Learning (ML) has rapidly evolved over the past few years and is transforming the landscape of business and technology. In this comprehensive article, we'll explore what machine learning is, its applications, and how it revolutionizes business operations. We will answer the fundamental questions about machine learning and provide insights into why this technology is essential for modern enterprises.
What is Machine Learning?
At its core, machine learning is a subset of artificial intelligence (AI). It is a technique that allows computers to learn from data and improve their performance over time without being explicitly programmed. Unlike traditional programming, where rules are defined manually, machine learning algorithms use statistical methods to identify patterns and make predictions based on input data.
Types of Machine Learning
Machine learning can generally be divided into three main types:
- Supervised Learning: In this type, the algorithm is trained on labeled datasets, meaning that both the input data and the correct output are provided. This approach is commonly used for classification and regression tasks.
- Unsupervised Learning: Here, the algorithm is given input data without labeled responses, and it seeks to find hidden patterns or intrinsic structures within the data. Clustering is a common example of unsupervised learning.
- Reinforcement Learning: This type involves training an agent to make a sequence of decisions by rewarding desired behaviors and/or punishing undesired ones. It is often used in gaming and robotics.
The Importance of Machine Learning in Business
Businesses across various sectors are leveraging the power of machine learning to enhance efficiency, improve customer experiences, and drive growth. Here are several reasons why machine learning has become indispensable:
1. Enhanced Decision-Making
Machine learning provides businesses with the analytical power to make data-driven decisions. By extracting insights from large datasets, companies can identify trends, optimize processes, and allocate resources more effectively.
2. Personalized Customer Experiences
One of the most impactful applications of machine learning is in creating personalized customer experiences. Retailers and service providers utilize machine learning algorithms to analyze customer data and tailor recommendations, promotions, and communications to individual preferences. This personalization increases customer satisfaction and loyalty.
3. Predictive Analytics
With machine learning, businesses can forecast future trends and behaviors. Predictive analytics driven by machine learning algorithms allows for enhanced inventory management, reduced operational costs, and improved sales forecasting. Organizations can proactively respond to changing market conditions and customer needs.
4. Operational Efficiency
By automating routine tasks and optimizing workflows, machine learning increases operational efficiency. Businesses can use ML algorithms to predict maintenance needs, manage supply chains, and streamline production scheduling. This efficiency leads to cost savings and better resource utilization.
5. Data-Driven Marketing Strategies
Machine learning enables advanced customer segmentation and targeting for marketing campaigns. By analyzing customer behavior and preferences, organizations can optimize their advertising strategies and maximize their marketing ROI.
Common Applications of Machine Learning
Machine learning's versatility means it can be applied in numerous fields. Some common applications include:
- Health Care: Predictive analytics can help in diagnosing diseases and managing patient data. Machine learning algorithms can analyze medical images with remarkable accuracy, aiding radiologists.
- Finance: Machine learning is crucial for fraud detection, risk assessment, and algorithmic trading. Financial institutions use ML to analyze transaction patterns and identify anomalies.
- Manufacturing: Predictive maintenance and quality control are enhanced through machine learning, allowing manufacturers to minimize downtime and improve product quality.
- Marketing: Companies leverage ML to understand customer behavior, automate A/B testing, and optimize their advertising spend through real-time analytics.
- Transportation: Machine learning is used in route optimization, demand forecasting, and self-driving vehicles, significantly impacting logistics and transportation industries.
Contemporary Trends in Machine Learning
The field of machine learning is ever-evolving, shaped by new technologies, methodologies, and applications. Some contemporary trends include:
1. AutoML (Automated Machine Learning)
AutoML simplifies the process of applying machine learning techniques by automating data preparation, model selection, and hyperparameter tuning. This democratizes access to machine learning capabilities, allowing non-experts to leverage its power.
2. Explainable AI (XAI)
As machine learning models become more complex, the need for transparency grows. Explainable AI focuses on making model decisions interpretable and understandable, which is crucial in sectors like finance and healthcare, where accountability is necessary.
3. Edge Computing
Combining machine learning with edge computing allows for real-time data processing and analysis closer to the source of data collection. This development enhances applications in IoT and has significant implications for speed and efficiency.
4. Federated Learning
Federated learning allows machine learning models to be trained across multiple decentralized devices holding local data, without transferring that data to a central server. This approach improves privacy and security, making it suitable for sensitive applications.
Challenges in Implementing Machine Learning
While machine learning presents numerous advantages, organizations also face challenges in its implementation:
- Data Quality: High-quality, relevant data is essential for effective machine learning. Businesses must invest in data cleaning and preprocessing to ensure accurate results.
- Skill Gaps: There is often a shortage of skilled professionals in machine learning and data science, making it difficult for organizations to build competent teams.
- Integration with Existing Systems: Integrating machine learning solutions with legacy systems can be a complex task requiring significant resources and expertise.
- Ethical Concerns: The use of machine learning raises ethical questions, especially in areas like bias, privacy, and transparency. Businesses must navigate these challenges carefully.
Conclusion: The Future of Machine Learning in Business
In summary, machine learning is a transformative technology that significantly impacts how businesses operate and strategize. Its applications span various sectors, providing enhanced decision-making, personalized experiences, and operational efficiencies.
As the landscape evolves, staying abreast of trends and preparing for challenges will be critical for organizations aiming to harness the full potential of machine learning. The broad adoption of this technology means that businesses must prioritize not just implementation but also ethical considerations and the capabilities of their workforce.
By embracing machine learning, businesses can unlock new opportunities, drive growth, and maintain a competitive edge in a rapidly changing market. The future is bright for machine learning, and those who invest in understanding and leveraging its capabilities will be well-positioned to thrive.
about machine learning